Analyzing survey data is a critical process that turns raw feedback into actionable insights, enabling businesses and researchers to make informed decisions. Whether you’re dealing with quantitative data, qualitative survey data, or a blend of both, understanding how to analyze survey data can reveal trends, patterns, and correlations that can shape strategies and drive success.
In this article, we’ll delve into the intricacies of survey data analysis, offering practical advice on analyzing survey results effectively, whether you’re handling customer surveys, research projects, or internal feedback initiatives.
Step 1: Collecting and Organizing Survey Data
Before diving into the complexities of analyzing survey data, the first step is to ensure that your data collection process is robust.
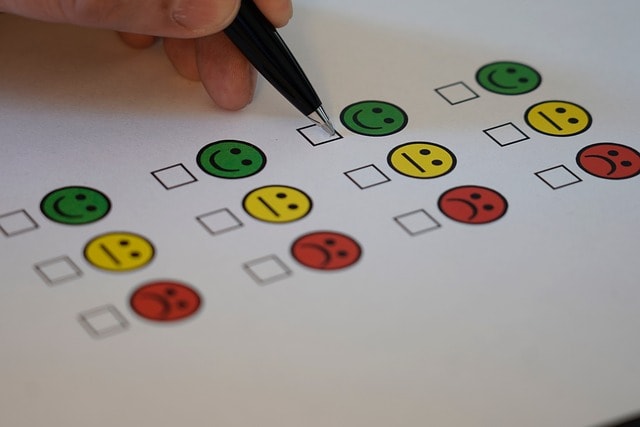
The quality of your survey data largely depends on the design of your survey questions, the method of data collection, and the accuracy of your survey sample. This is especially true for both quantitative and qualitative research, where data integrity is crucial for drawing meaningful conclusions.
In the initial phase of survey data collection, ensure that the questions are clearly defined and aligned with your research question. Consider the type of data you are collecting—whether it’s numerical data through interval or ratio scales, or open-ended responses in qualitative surveys. For accurate survey data analysis, focus on minimizing biases during survey design.
You must also ensure that the sample size is statistically significant to produce meaningful results, which is critical in both quantitative data analysis and qualitative research.
Step 2: Preparing Survey Data for Analysis
Once the survey data is collected, the next step is to prepare it for analysis. This involves cleaning and organizing the raw data, ensuring that all survey responses are recorded accurately. Common issues, such as missing data points, duplicate responses, or outliers, need to be addressed during this phase.
For quantitative data, numerical values should be organized into a structured format, usually in a spreadsheet or within a software tool designed for survey data analysis. This makes it easier to conduct statistical analysis and draw meaningful conclusions.
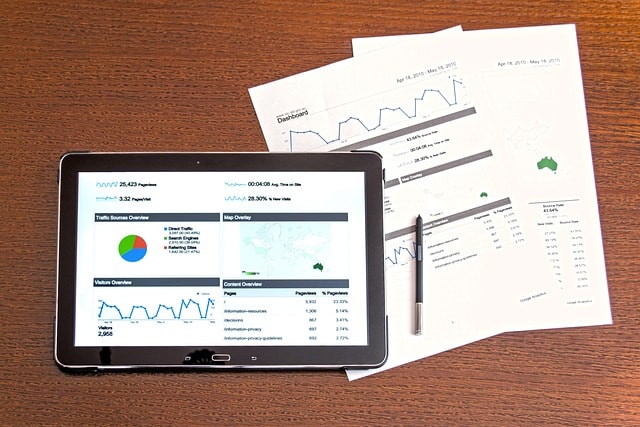
In cases where qualitative survey data has been collected, such as open-ended responses, organizing the data into categories or themes will help during the analysis phase.
To make the data analysis process smoother, survey data can be segmented based on demographic data, survey questions, or any other variable that is crucial to your research. This segmentation allows for more granular analysis and helps identify trends that may be hidden when looking at all the data collectively.
Step 3: Analyzing Quantitative Survey Data
Quantitative survey data analysis is largely focused on numerical data and typically involves statistical analysis methods. The goal is to uncover patterns, correlations, and trends that can inform business decisions or answer specific research questions.
Statistical analysis of survey data can range from simple calculations, such as averages and percentages, to more complex techniques, such as regression analysis and cross-tabulation.
Regression analysis is particularly useful when you’re trying to understand the relationship between two or more independent variables and their effect on a dependent variable.
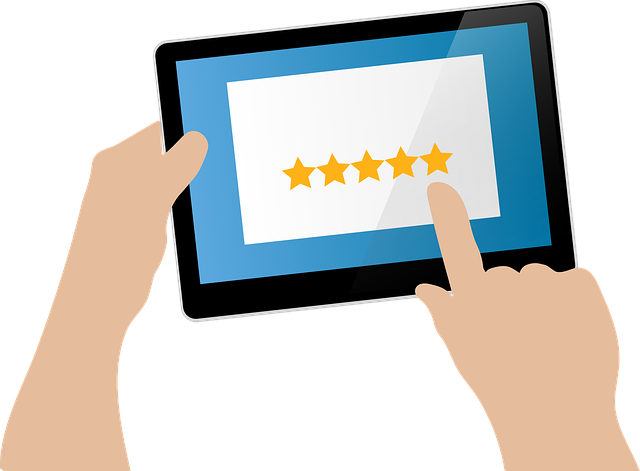
Another important aspect of analyzing quantitative data is determining statistical significance. This helps in identifying whether the patterns observed in the data are due to chance or if they are statistically significant. Analyzing survey data with statistical significance in mind ensures that your conclusions are based on reliable data, increasing the credibility of your findings.
In addition to standard statistical techniques, machine learning can also be used to analyze large datasets and uncover hidden insights. This is particularly useful when dealing with online surveys or other data sources where the volume of data collected is too large for manual analysis.
Step 4: Analyzing Qualitative Survey Data
While quantitative data provides numerical insights, qualitative data offers a deeper understanding of the emotions, thoughts, and motivations behind the survey responses. Analyzing qualitative survey data requires a different approach compared to numerical data.
For qualitative survey data analysis, start by reviewing all open-ended responses to identify recurring themes or patterns. This could involve coding the data, where each response is categorized into a particular theme or sentiment.
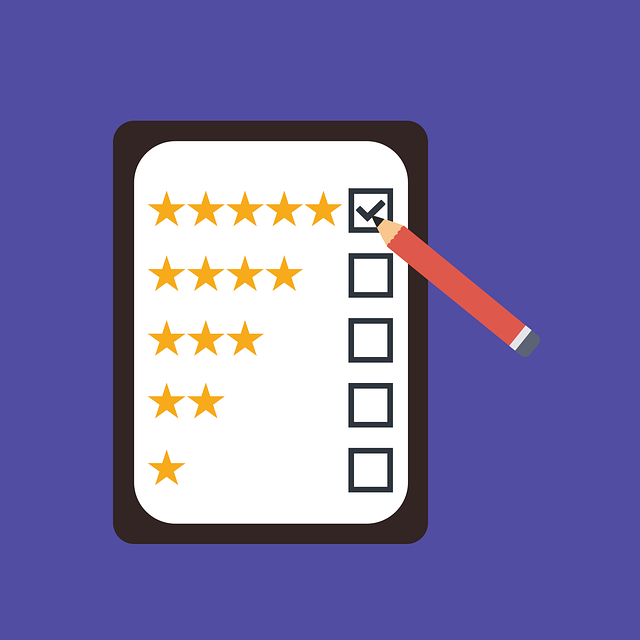
These themes can then be quantified to provide a broader overview of the sentiments expressed by the survey respondents.
Focus groups and interviews are also excellent sources of qualitative data, providing further insights into customer satisfaction, employee feedback, or other areas of interest. Analyzing data from these sources often requires transcribing the discussions and identifying key insights from the conversations.
When analyzing qualitative data, it’s important to recognize that it complements quantitative data rather than replacing it. By combining both forms of data, you can draw meaningful conclusions that are supported by numbers while also understanding the underlying motivations and attitudes of your respondents.
Step 5: Presenting Survey Data
Once the survey data has been analyzed, the next step is to present your findings. The way you present survey data will depend on your audience and the key insights you want to highlight. Visual representations, such as pie charts, bar graphs, and cross-tabulation tables, are useful for conveying quantitative data in a clear and concise manner.
When presenting qualitative data, consider using direct quotes from respondents or creating word clouds to illustrate common themes. This adds depth to your report and helps bring the data to life for your audience.
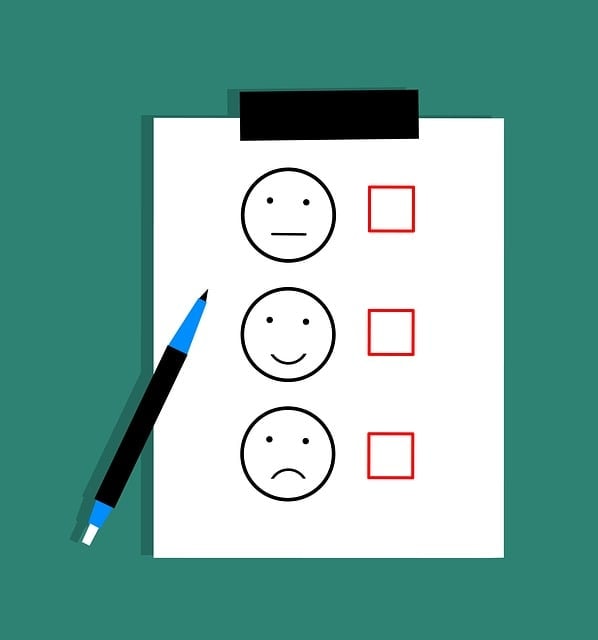
Your survey report should clearly outline the research questions, methods used for data analysis, and the key findings. Be sure to explain how the data collected supports your conclusions, and where appropriate, suggest actionable insights based on the results.
When presenting your data, also consider whether longitudinal analysis is relevant. If you’ve collected data over time, showing trends and changes can provide a valuable perspective that one-time snapshots can’t offer. This is particularly useful when tracking customer satisfaction or monitoring the effectiveness of ongoing initiatives.
Step 6: Drawing Meaningful Conclusions from Survey Data
The ultimate goal of analyzing survey data is to draw meaningful conclusions that can drive decisions. This step involves interpreting your findings, understanding the implications, and making informed recommendations.
For quantitative data, consider how the survey results can be used to improve processes, products, or customer experiences. For qualitative data, look at how the sentiments expressed by respondents can shape future strategies.
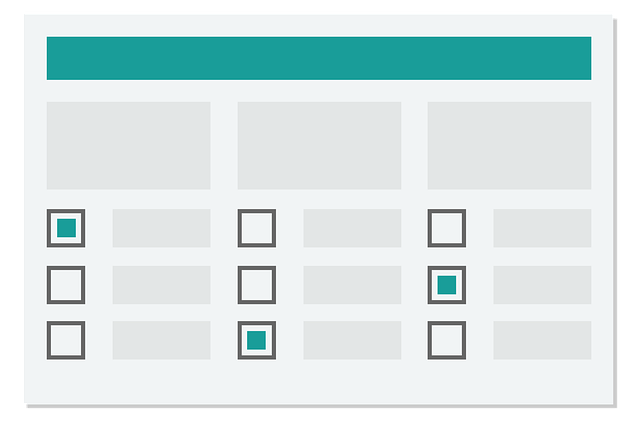
Whether your focus is on customer insights, employee feedback, or market research, the conclusions you draw should be actionable and based on solid data analysis methods.
Remember to consider the limitations of your survey data. Not all data points will be equally relevant, and not every trend identified will be statistically significant. Being aware of these limitations will allow you to draw conclusions that are realistic and grounded in the data.
Step 7: Continuous Improvement through Survey Data Analysis
Survey data analysis is not a one-time process; it should be a continuous effort to track data, analyze trends, and refine strategies based on the findings. As you analyze past data and collect new survey responses, you can compare the results to identify shifts in attitudes, preferences, or behaviors over time.
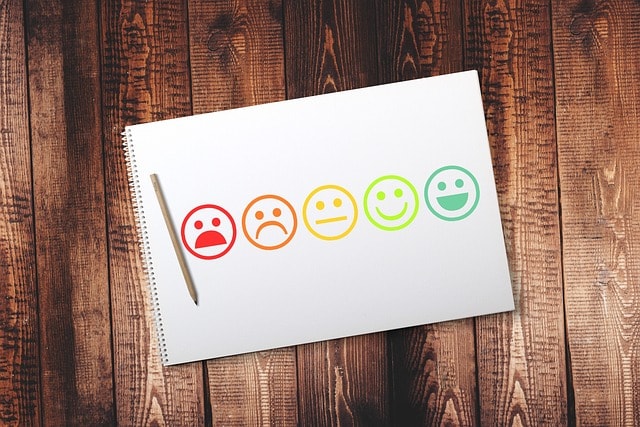
Continuous analysis also allows you to refine your survey design and data analysis methods. As you become more familiar with your data and the insights it provides, you can ask more targeted survey questions and apply more advanced analysis techniques.
By effectively analyzing all your data, you can turn survey results into a powerful tool for ongoing improvement, ensuring that your business or research remains responsive to the needs and preferences of your target audience.
Myth Busting: Common Misconceptions in Survey Data Analysis
Survey data analysis is often surrounded by misconceptions that can mislead researchers and businesses.
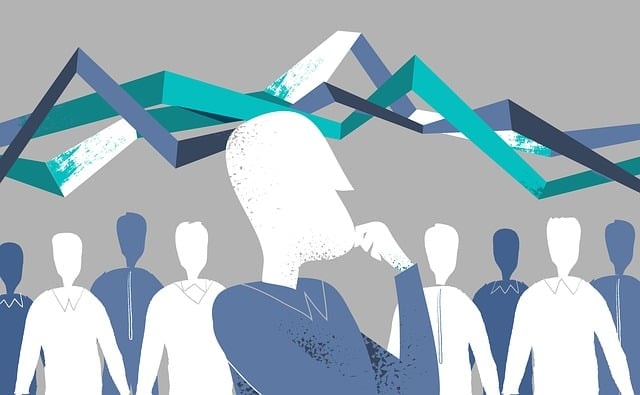
Let’s debunk five common myths and clarify the facts:
Myth 1: All survey data is equally valuable.
Reality: Not all data points are relevant. The quality of survey data depends on how well the survey questions align with research objectives. Poorly designed surveys can lead to misleading insights, even with a large dataset.
Myth 2: You need a massive sample size to analyze survey results effectively.
Reality: While larger sample sizes can increase accuracy, determining sample size should be based on achieving statistical significance, not sheer numbers. Smaller, well-targeted samples can still yield valuable insights.
Myth 3: Open-ended questions are too difficult to analyze.
Reality: Analyzing open-ended responses is becoming easier with advances in text analysis tools. These questions provide qualitative insights that can complement quantitative data.
Myth 4: You can skip data cleaning if your survey tool is advanced.
Reality: Even the most advanced survey tools require data cleaning. Ensuring accuracy in the data collected is crucial for producing reliable survey results analysis.
Myth 5: Presenting survey results is just about showing numbers.
Reality: Effective presentation goes beyond numbers. Visualizations, qualitative insights, and clear narratives help transform raw data into actionable insights for decision-makers.
Expert Comment by Dr. Michael Lawson, Ph.D.
Renowned Data Scientist and Survey Analysis Expert
“Survey analysis requires a deep understanding of both quantitative and qualitative data. The key to effective survey data analysis lies in the methods chosen to analyze survey results.
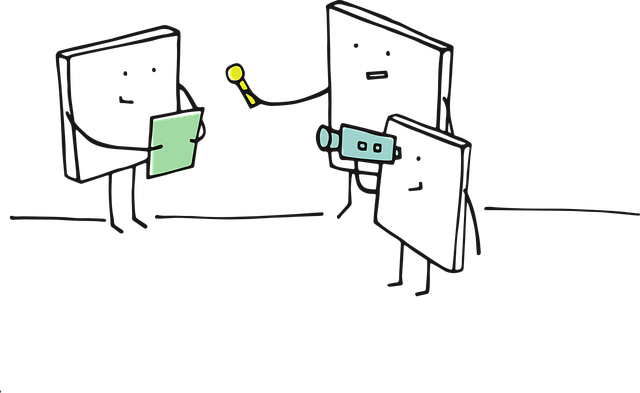
For example, ensuring that all the survey data is properly cleaned and organized is crucial before diving into more complex statistical analysis.
When interpreting research findings, it’s essential to verify that your survey is based on statistically significant research, which often means carefully determining your sample size in advance.
When working with dependent variables, understanding how many respondents you need to achieve statistical power is vital. Survey results analysis can be greatly affected by how well you’ve controlled for potential confounding factors, such as a third variable that could influence the relationship between two independent variables. This is why it’s critical to use robust survey data analysis methods.
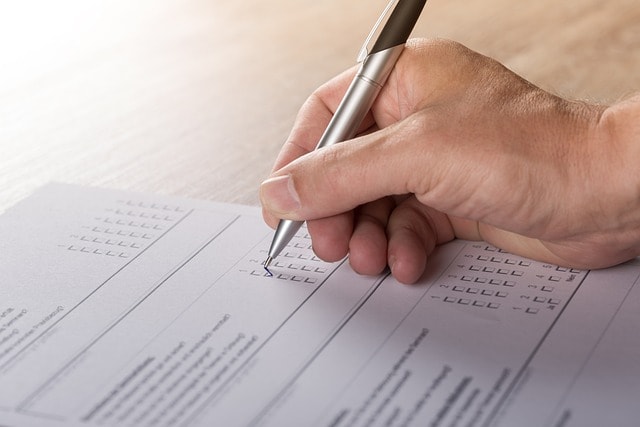
Furthermore, when analyzing open-ended responses, applying thematic coding techniques can help you make sense of qualitative data. Similarly, understanding the nuances of interval scales can help when working with quantitative data. In customer feedback surveys, presenting survey results clearly is as important as collecting data accurately.
The same data can yield different insights depending on how it’s analyzed and presented, so a holistic approach to survey analysis is always recommended.”
Insider Tips: Expert Strategies for Survey Data Analysis
Here are five exclusive tips from industry professionals to elevate your survey data analysis:
1. Pre-Test Your Survey Questions
Before launching your survey, conduct a pilot test with a small group. This allows you to identify confusing questions or potential biases in your survey design, ensuring that the data collected is reliable and aligned with your research objectives.
2. Use Weighting for Representative Data
If your survey sample isn’t perfectly representative of your target population, apply weighting to adjust for demographic imbalances. This advanced technique ensures that your survey analysis reflects the broader population more accurately.
3. Segment Your Data for Deeper Insights
Don’t just analyze the overall data—segment it by demographics, behaviors, or other key factors. This allows you to identify trends within specific subgroups of survey respondents, uncovering hidden insights that may be missed in aggregate data analysis.
4. Automate Open-Ended Response Analysis
Use text analysis tools to automatically code and categorize open-ended responses. These tools speed up the process and help ensure consistency, allowing you to extract qualitative insights more efficiently while reducing human bias in interpretation.
5. Combine Longitudinal and Cross-Sectional Data
When possible, incorporate both longitudinal and cross-sectional analysis in your survey analysis. Longitudinal data reveals trends over time, while cross-sectional data provides snapshots of specific moments. Combining both offers a comprehensive view of your survey results and helps draw meaningful conclusions.
Future Implications
As the landscape of survey analysis continues to evolve, future trends will likely emphasize even more sophisticated methods to collect data and analyze survey results.
With the rise of AI and machine learning, tools to analyze open-ended responses will become more advanced, offering faster and more accurate insights.
Additionally, determining sample size will become increasingly complex as researchers aim to balance precision with cost-effectiveness in a world of ever-expanding datasets.
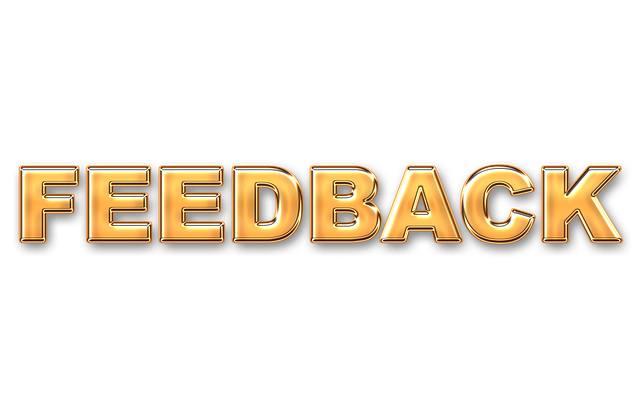
The challenge of effectively presenting survey results will grow as data visualization tools strive to keep pace with increasingly large and multifaceted datasets.
Innovations in survey design, particularly in crafting open-ended questions, will likely push boundaries, seeking to elicit more meaningful responses while ensuring that data remains manageable. These developments will require experts to stay agile, continuously adapting to new tools and methodologies in survey data analysis – present survey results and determine sample size.
Leveraging Software Tools for Survey Data Analysis
In today’s digital landscape, software tools are essential for efficiently analyzing survey data. From basic platforms like Excel to specialized survey analysis software, these tools simplify the process of transforming raw data into actionable insights. Advanced survey data analysis tools often come with built-in statistical analysis features, making it easier to identify trends, calculate statistical significance, and analyze open-ended responses.
When working with quantitative data, software can automate tasks like cross-tabulation and regression analysis, saving time and reducing errors. Many tools also offer data visualization features, allowing you to present survey results in a clear and compelling way. Visualizing data points with charts and graphs not only helps in identifying trends but also makes your survey report more accessible to stakeholders.
For qualitative data, tools that analyze open-ended responses, such as text analysis software, can quickly categorize responses into themes or sentiments. This speeds up the process of drawing meaningful conclusions from qualitative survey data, ensuring that no valuable insights are overlooked. By leveraging the right software tools, you can streamline your data analysis process, improve accuracy, and deliver more impactful survey results. So, how about you give it a go, now and today?
Conclusion
Learning how to analyze survey data is essential for turning raw responses into valuable insights that can inform decisions, shape strategies, and drive success. Whether you’re working with quantitative or qualitative data, the process involves careful preparation, thorough analysis, and thoughtful presentation. By focusing on statistical analysis, identifying trends, and drawing meaningful conclusions, you can leverage survey data to achieve your goals.
Through continuous improvement and ongoing analysis, you’ll ensure that every piece of feedback collected through your surveys contributes to your understanding of your audience, your business, or your research subject. In the end, it’s not just about collecting data—it’s about using it to make better decisions.
FAQ
What is survey data?
Survey data consists of responses collected from a set of questions designed to gather information from a target audience. This data can be both quantitative (numerical) and qualitative (textual), serving as the foundation for survey analysis to draw meaningful conclusions.
What is survey analysis?
Survey analysis is the process of examining survey data to extract insights, identify trends, and answer research questions. It involves both quantitative data analysis, such as statistical analysis, and qualitative data analysis, like analyzing open-ended responses.
How do I start analyzing survey data?
Start by organizing and cleaning the survey data, ensuring accuracy. Then, identify the research questions you’re trying to answer. Apply appropriate data analysis methods, such as statistical analysis for quantitative data and thematic coding for qualitative survey data, to draw meaningful conclusions.
What is quantitative data in surveys?
Quantitative data refers to numerical information gathered through survey responses, typically from closed-ended questions. This data can be used for statistical analysis to identify trends, measure responses, and provide measurable insights.
Why is statistical analysis important in survey analysis?
Statistical analysis helps uncover patterns, relationships, and significance in quantitative data. It allows researchers to draw meaningful conclusions from survey data, ensuring that findings are based on evidence rather than random variation.
How do I identify trends in survey data?
To identify trends, analyze the survey data over time or across different segments of survey respondents. Use statistical methods, such as regression analysis or cross-tabulation, to highlight patterns that can inform future decisions and strategies.
What should be included in a survey report?
A survey report should include an overview of the research questions, data analysis methods used, key findings, and actionable insights. Present both quantitative data and qualitative data clearly, using visuals and narrative explanations to convey conclusions effectively.
What is qualitative data in surveys?
Qualitative data in surveys comes from open-ended responses, offering insights into thoughts, opinions, and motivations. Unlike quantitative data, it’s not numerical but provides context and depth to the survey analysis, helping to understand the “why” behind the numbers.
How can survey respondents’ answers impact survey analysis?
Survey respondents’ answers are the raw material for survey analysis. Their quality and honesty directly affect the data collected, and thus, the conclusions drawn. Careful question design and appropriate data analysis ensure that respondents’ insights lead to meaningful conclusions.
What does statistically significant mean in survey data analysis?
Statistically significant results indicate that the patterns observed in the data are unlikely to have occurred by chance. In survey data analysis, achieving statistical significance means that the findings are reliable and can be generalized to a larger population.
How should I analyze open-ended responses?
To analyze open-ended responses, categorize and code the qualitative data into themes or patterns. This thematic analysis helps identify commonalities or unique insights that can complement quantitative data, providing a more holistic view of the survey results.
What role do research questions play in survey analysis?
Research questions guide the entire survey analysis process. They help determine what data to collect, which analysis methods to use, and ultimately shape the conclusions drawn. Clear research questions ensure that the survey analysis remains focused and relevant.
How can I draw meaningful conclusions from survey data?
To draw meaningful conclusions, ensure that your data analysis methods are robust, and your findings align with the research questions. Use statistical analysis for quantitative data and thematic analysis for qualitative data to interpret the survey data accurately.
What are the benefits of using open-ended questions in surveys?
Open-ended questions allow survey respondents to express their thoughts freely, providing rich qualitative data. This data can reveal insights that structured questions might miss, offering a deeper understanding of attitudes, preferences, and motivations.
What is regression analysis in survey data analysis?
Regression analysis is a statistical method used to examine the relationship between one dependent variable and one or more independent variables. In survey data analysis, it helps predict outcomes and understand how different factors influence survey responses.
How can I ensure my survey sample size is statistically significant?
To ensure a statistically significant sample size, calculate the minimum number of respondents needed based on the desired confidence level and margin of error. A larger, well-targeted sample increases the reliability of your survey results and ensures that they are generalizable.
How do I handle missing data in survey analysis?
Missing data can be addressed by using methods such as data imputation, where missing values are estimated, or by excluding incomplete responses. Carefully handling missing data ensures the accuracy of your survey analysis and helps draw meaningful conclusions.
How should I present survey results?
Present survey results clearly and concisely, using visuals like charts or graphs for quantitative data and summaries or quotes for qualitative data. Tailor your presentation to your audience, ensuring that the key findings and actionable insights are easy to understand.
What are interval scales in survey data?
Interval scales measure variables with equal intervals between values but lack a true zero point (e.g., temperature in Celsius). In survey data, they allow for meaningful comparisons between responses, facilitating more precise data analysis.
Why is data cleaning important in survey analysis?
Data cleaning ensures that the survey data is accurate and free from errors such as duplicate entries or inconsistent responses. Properly cleaned data is essential for reliable data analysis, as it ensures that the conclusions drawn are based on valid and consistent information.
Comments are closed